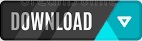
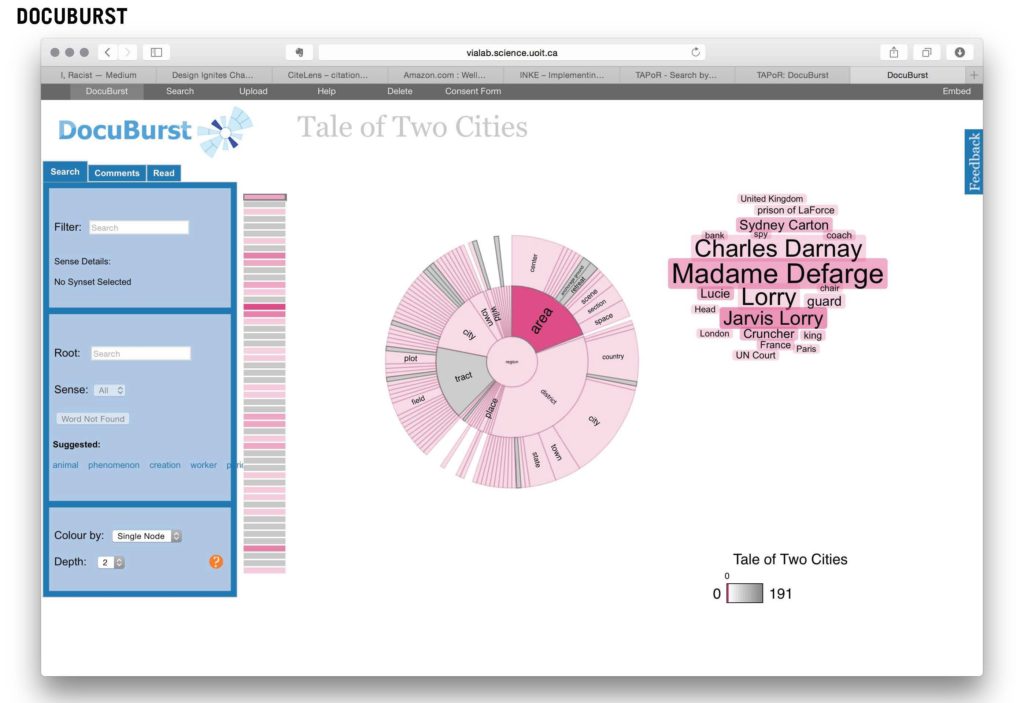
I worked with industry partners and the ADAPT Research Centre on recommender systems and released large-scale datasets to facilitate the research on recommender-systems including Docear, RARD, and DonorsChoose. I have worked in the field of recommender systems for more than a decade, and developed several recommender systems myself including Docear, Mr. This opens the door for interdisciplinary cooperation with exciting challenges and high potential for impactful work. Recommender-systems research can also be conducted in almost every domain including e-commerce, movies, music, art, health, food, legal, or finance.

information retrieval, natural language processing, graphic and user interface design, machine learning, distributed computing, high performance computing) the social sciences, and many more. Given this broad range, many disciplines contribute to recommender-systems research including computer science (e.g. The areas of interest range from hard mathematical/algorithmic problems over user-centric problems (user interfaces, evaluations, privacy) to ethical and political questions (bias, information bubbles). the most often read/shared/liked news) or items with the highest overall user rating.įrom a research perspective, recommender-systems are one of the most diverse areas imaginable. Sometimes, even very simple recommendation approaches perform well, such as recommending the most popular items (e.g. Those movies that user A liked but that user B had not yet watched would then be recommended to user B, and vice versa. For instance, if two users, A and B, both watched the same movies and rated them similarly, the two users would be considered like-minded. With Collaborative Filtering users are grouped by their taste where taste is usually measured by the similarity of their ratings for some items. For instance, if a user reads an article in which the term “Machine Learning” occurs, then other articles that also contain that term would then be recommended. In Content-Based Filtering, items with similar content are recommended based on items a user previously interacted with. There are two main approaches to recommender systems: Content-Based Filtering (CBF) and Collaborative Filtering (CF). Other studies of recommender systems also consistently report increases of several percentages in page views, time spent on page and revenue. Netflix reports that its recommender system influences users’ choice for 80% of watched movies, and the recommender system contributes more than $1B per year to its business. For instance, Amazon’s recommender system increases sales by around 8%. Sometimes, recommender systems ‘only’ provide some added value for customers and improve the user experience, but recommender systems are often a central part of a company’s business model. Hardly any enterprise could afford to ignore personalization techniques and not have a recommender system.
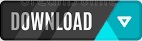